In-Silico Charakterization of disease-associated sites in ion channel and transporter proteins
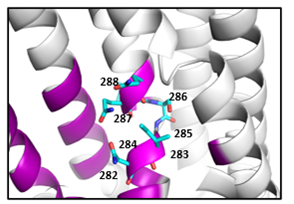
We are proposing the development of an in-silico ion channel specific variant
assessment and classification framework which subsequently will allow diagnostically
meaningful variant prediction and identification of therapeutic target sites at ion channel
proteins. Overall, we will address the following three specific aims:
1) Development of a comprehensive ion channel variant knowledge repository. We will
collect and curate all publicly, commercially and in associated consortia available genetic variant data, experimentally solved protein structures and homology models as well as RNA expression signatures for neuronal ion channel proteins and transporters. To benefit from expert knowledge and to validate our predictions with experimental data we will focus on ion channel proteins and their gene families studied in the other projects such as the voltage-gated calcium (CACNA1 family), sodium (SCN family, and the potassium channels (KCN family).
2) Development of a novel gene-family based prediction method for variant interpretation. Based on an improved version of our recently developed method (Lal et al., 2020 Genome Medicine) , gene family conservation information of ion channel proteins will be leveraged to identify amino acids under evolutionary constraint. We will incorporate additional features in the classifier such as an amino acid chemical exchange score, protein 3D structure knowledge, and protein sub-domain information.
3) Statistical correlation of patient variants on protein domains and patient phenotypes to
unlock phenotypic heterogeneity across patients. We will re-evaluate known and newly
discovered variants together with patient phenotype data provided by the central phenotype
repository of the project and other members of the consortium and we also will make suggestions for potentially new variant-causing variants with focus on the genes studied in the other projects. For each channelopathy, we will group the complex patient phenotypes into clinical symptom-based subgroups and correlate their variant localization on the protein sequence and structure.